PyTorch模型轉(zhuǎn)TensorRT是怎么實現(xiàn)的?
ubuntu-18.04PyTorch-1.8.1onnx-1.9.0onnxruntime-1.7.2cuda-11.1cudnn-8.2.0TensorRT-7.2.3.4PyTorch轉(zhuǎn)ONNX
Step1:安裝ONNX和ONNXRUNTIME
網(wǎng)上找到的安裝方式是通過pip
pip install onnxpip install onnxruntime
如果使用的是Anaconda環(huán)境,conda安裝也是可以的。
conda install -c conda-forge onnxconda install -c conda-forge onnxruntime
Step2:安裝netron
netron是用于可視化網(wǎng)絡結(jié)構的,便于debug。
pip install netron
Step3 PyTorch轉(zhuǎn)ONNx
安裝完成后,可以根據(jù)下面code進行轉(zhuǎn)換。
#--*-- coding:utf-8 --*--import onnx # 注意這里導入onnx時必須在torch導入之前,否則會出現(xiàn)segmentation faultimport torchimport torchvision from model import Netmodel= Net(args).cuda()#初始化模型checkpoint = torch.load(checkpoint_path)net.load_state_dict(checkpoint[’state_dict’])#載入訓練好的權重文件print ('Model and weights LOADED successfully')export_onnx_file = ’./net.onnx’x = torch.onnx.export(net,torch.randn(1,1,224,224,device=’cuda’), #根據(jù)輸入要求初始化一個dummy inputexport_onnx_file,verbose=False, #是否以字符串形式顯示計算圖input_names = ['inputs']+['params_%d'%i for i in range(120)],#輸入節(jié)點的名稱,這里也可以給一個list,list中名稱分別對應每一層可學習的參數(shù),便于后續(xù)查詢output_names = ['outputs'],# 輸出節(jié)點的名稱opset_version = 10,#onnx 支持采用的operator set, 應該和pytorch版本相關do_constant_folding = True,dynamic_axes = {'inputs':{0:'batch_size'}, 2:'h', 3:'w'}, 'outputs':{0: 'batch_size'},})net = onnx.load(’./erfnet.onnx’) #加載onnx 計算圖onnx.checker.check_model(net) # 檢查文件模型是否正確onnx.helper.printable_graph(net.graph) #輸出onnx的計算圖
dynamic_axes用于指定輸入、輸出中的可變維度。輸入輸出的batch_size在這里都設為了可變,輸入的第2和第3維也設置為了可變。
Step 4:驗證ONNX模型
下面可視化onnx模型,同時測試模型是否正確運行
import netronimport onnxruntimeimport numpy as npfrom PIL import Imageimport cv2netron.start(’./net.onnx’)test_image = np.asarray(Image.open(test_image_path).convert(’L’),dtype=’float32’) /255.test_image = cv2.resize(np.array(test_image),(224,224),interpolation = cv2.INTER_CUBIC)test_image = test_image[np.newaxis,np.newaxis,:,:]session = onnxruntime.InferenceSession(’./net.onnx’)outputs = session.run(None, {'inputs': test_image})print(len(outputs))print(outputs[0].shape)#根據(jù)需要處理一下outputs[0],并可視化一下結(jié)果,看看結(jié)果是否正常ONNX轉(zhuǎn)TensorRT
Step1:從NVIDIA下載TensorRT下載安裝包 https://developer.nvidia.com/tensorrt
根據(jù)自己的cuda版本選擇,我選擇的是TensorRT 7.2.3,下載到本地。
cd download_pathdpkg -i nv-tensorrt-repo-ubuntu1804-cuda11.1-trt7.2.3.4-ga-20210226_1-1_amd64.debsudo apt-get updatesudo apt-get install tensorrt
查了一下NVIDIA的官方安裝教程https://docs.nvidia.com/deeplearning/tensorrt/quick-start-guide/index.html#install,由于可能需要調(diào)用TensorRT Python API,我們還需要先安裝PyCUDA。這邊先插入一下PyCUDA的安裝。
pip install ’pycuda<2021.1’
遇到任何問題,請參考官方說明 https://wiki.tiker.net/PyCuda/Installation/Linux/#step-1-download-and-unpack-pycuda如果使用的是Python 3.X,再執(zhí)行一下以下安裝。
sudo apt-get install python3-libnvinfer-dev
如果需要ONNX graphsurgeon或使用Python模塊,還需要執(zhí)行以下命令。
sudo apt-get install onnx-graphsurgeon
驗證是否安裝成功。
dpkg -l | grep TensorRT
得到類似上圖的結(jié)果就是安裝成功了。
問題:此時在python中import tensorrt,得到ModuleNotFoundError: No module named ’tensorrt’的報錯信息。
網(wǎng)上查了一下,通過dpkg安裝的tensorrt是默認安裝在系統(tǒng)python中,而不是Anaconda環(huán)境的python里的。由于系統(tǒng)默認的python是3.6,而Anaconda里使用的是3.8.8,通過export PYTHONPATH的方式,又會出現(xiàn)python版本不匹配的問題。
重新搜索了一下如何在anaconda環(huán)境里安裝tensorRT。
pip3 install --upgrade setuptools pippip install nvidia-pyindexpip install nvidia-tensorrt
驗證一下這是Anconda環(huán)境的python是否可以import tensorrt。
import tensorrtprint(tensorrt.__version__)#輸出8.0.0.3
Step 2:ONNX轉(zhuǎn)TensorRT
先說一下,在這一步里遇到了*** AttributeError: ‘tensorrt.tensorrt.Builder’ object has no attribute ’max_workspace_size’的報錯信息。網(wǎng)上查了一下,是8.0.0.3版本的bug,要退回到7.2.3.4。emmm…
pip unintall nvidia-tensorrt #先把8.0.0.3版本卸載掉pip install nvidia-tensorrt==7.2.* --index-url https://pypi.ngc.nvidia.com # 安裝7.2.3.4banben
轉(zhuǎn)換代碼
import pycuda.autoinit import pycuda.driver as cudaimport tensorrt as trtimport torch import time from PIL import Imageimport cv2,osimport torchvision import numpy as npfrom scipy.special import softmax### get_img_np_nchw h和postprocess_the_output函數(shù)根據(jù)需要進行修改TRT_LOGGER = trt.Logger()def get_img_np_nchw(img_path):img = Image.open(img_path).convert(’L’)img = np.asarray(img, dtype=’float32’)img = cv2.resize(np.array(img),(224, 224), interpolation = cv2.INTER_CUBIC)img = img / 255.img = img[np.newaxis, np.newaxis]return imageclass HostDeviceMem(object): def __init__(self, host_mem, device_mem):'''host_mom指代cpu內(nèi)存,device_mem指代GPU內(nèi)存'''self.host = host_memself.device = device_mem def __str__(self):return 'Host:n' + str(self.host) + 'nDevice:n' + str(self.device) def __repr__(self):return self.__str__()def allocate_buffers(engine): inputs = [] outputs = [] bindings = [] stream = cuda.Stream() for binding in engine:size = trt.volume(engine.get_binding_shape(binding)) * engine.max_batch_sizedtype = trt.nptype(engine.get_binding_dtype(binding))# Allocate host and device buffershost_mem = cuda.pagelocked_empty(size, dtype)device_mem = cuda.mem_alloc(host_mem.nbytes)# Append the device buffer to device bindings.bindings.append(int(device_mem))# Append to the appropriate list.if engine.binding_is_input(binding): inputs.append(HostDeviceMem(host_mem, device_mem))else: outputs.append(HostDeviceMem(host_mem, device_mem)) return inputs, outputs, bindings, streamdef get_engine(max_batch_size=1, onnx_file_path='', engine_file_path='',fp16_mode=False, int8_mode=False,save_engine=False): ''' params max_batch_size: 預先指定大小好分配顯存 params onnx_file_path: onnx文件路徑 params engine_file_path: 待保存的序列化的引擎文件路徑 params fp16_mode: 是否采用FP16 params int8_mode: 是否采用INT8 params save_engine: 是否保存引擎 returns: ICudaEngine ''' # 如果已經(jīng)存在序列化之后的引擎,則直接反序列化得到cudaEngine if os.path.exists(engine_file_path):print('Reading engine from file: {}'.format(engine_file_path))with open(engine_file_path, ’rb’) as f, trt.Runtime(TRT_LOGGER) as runtime: return runtime.deserialize_cuda_engine(f.read()) # 反序列化 else: # 由onnx創(chuàng)建cudaEngine# 使用logger創(chuàng)建一個builder # builder創(chuàng)建一個計算圖 INetworkDefinitionexplicit_batch = 1 << (int)(trt.NetworkDefinitionCreationFlag.EXPLICIT_BATCH)# In TensorRT 7.0, the ONNX parser only supports full-dimensions mode, meaning that your network definition must be created with the explicitBatch flag set. For more information, see Working With Dynamic Shapes.with trt.Builder(TRT_LOGGER) as builder, builder.create_network(explicit_batch) as network, trt.OnnxParser(network, TRT_LOGGER) as parser, builder.create_builder_config() as config: # 使用onnx的解析器綁定計算圖,后續(xù)將通過解析填充計算圖 profile = builder.create_optimization_profile() profile.set_shape('inputs', (1, 1, 224, 224),(1,1,224,224),(1,1,224,224)) config.add_optimization_profile(profile) config.max_workspace_size = 1<<30 # 預先分配的工作空間大小,即ICudaEngine執(zhí)行時GPU最大需要的空間 builder.max_batch_size = max_batch_size # 執(zhí)行時最大可以使用的batchsize builder.fp16_mode = fp16_mode builder.int8_mode = int8_mode if int8_mode:# To be updatedraise NotImplementedError # 解析onnx文件,填充計算圖 if not os.path.exists(onnx_file_path):quit('ONNX file {} not found!'.format(onnx_file_path)) print(’loading onnx file from path {} ...’.format(onnx_file_path)) # with open(onnx_file_path, ’rb’) as model: # 二值化的網(wǎng)絡結(jié)果和參數(shù) # print('Begining onnx file parsing') # parser.parse(model.read()) # 解析onnx文件 parser.parse_from_file(onnx_file_path) # parser還有一個從文件解析onnx的方法 print('Completed parsing of onnx file') # 填充計算圖完成后,則使用builder從計算圖中創(chuàng)建CudaEngine print('Building an engine from file{}’ this may take a while...'.format(onnx_file_path)) ################# # import pdb;pdb.set_trace() print(network.get_layer(network.num_layers-1).get_output(0).shape) # network.mark_output(network.get_layer(network.num_layers -1).get_output(0)) engine = builder.build_engine(network,config) # 注意,這里的network是INetworkDefinition類型,即填充后的計算圖 print('Completed creating Engine') if save_engine: #保存engine供以后直接反序列化使用with open(engine_file_path, ’wb’) as f: f.write(engine.serialize()) # 序列化 return enginedef do_inference(context, bindings, inputs, outputs, stream, batch_size=1): # Transfer data from CPU to the GPU. [cuda.memcpy_htod_async(inp.device, inp.host, stream) for inp in inputs] # Run inference. context.execute_async(batch_size=batch_size, bindings=bindings, stream_handle=stream.handle) # Transfer predictions back from the GPU. [cuda.memcpy_dtoh_async(out.host, out.device, stream) for out in outputs] # Synchronize the stream stream.synchronize() # Return only the host outputs. return [out.host for out in outputs]def postprocess_the_outputs(outputs, shape_of_output): outputs = outputs.reshape(*shape_of_output) out = np.argmax(softmax(outputs,axis=1)[0,...],axis=0) # import pdb;pdb.set_trace() return out# 驗證TensorRT模型是否正確onnx_model_path = ’./Net.onnx’max_batch_size = 1# These two modes are dependent on hardwaresfp16_mode = Falseint8_mode = Falsetrt_engine_path = ’./model_fp16_{}_int8_{}.trt’.format(fp16_mode, int8_mode)# Build an engineengine = get_engine(max_batch_size, onnx_model_path, trt_engine_path, fp16_mode, int8_mode , save_engine=True)# Create the context for this enginecontext = engine.create_execution_context()# Allocate buffers for input and outputinputs, outputs, bindings, stream = allocate_buffers(engine) # input, output: host # bindings# Do inferenceimg_np_nchw = get_img_np_nchw(img_path)inputs[0].host = img_np_nchw.reshape(-1)shape_of_output = (max_batch_size, 2, 224, 224)# inputs[1].host = ... for multiple inputt1 = time.time()trt_outputs = do_inference(context, bindings=bindings, inputs=inputs, outputs=outputs, stream=stream) # numpy datat2 = time.time()feat = postprocess_the_outputs(trt_outputs[0], shape_of_output)print(’TensorRT ok’)print('Inference time with the TensorRT engine: {}'.format(t2-t1))
根據(jù)https://www.jb51.net/article/187266.htm文章里的方法,轉(zhuǎn)換的時候會報下面的錯誤:
原來我是根據(jù)鏈接里的代買進行轉(zhuǎn)換的,后來進行了修改,按我文中的轉(zhuǎn)換代碼不會有問題,
修改的地方在:
with trt.Builder(TRT_LOGGER) as builder, builder.create_network(explicit_batch) as network, trt.OnnxParser(network, TRT_LOGGER) as parser, builder.create_builder_config() as config: # 使用onnx的解析器綁定計算圖,后續(xù)將通過解析填充計算圖 profile = builder.create_optimization_profile() profile.set_shape('inputs', (1, 1, 224, 224),(1,1,224,224),(1,1,224,224)) config.add_optimization_profile(profile) config.max_workspace_size = 1<<30 # 預先分配的工作空間大小,即ICudaEngine執(zhí)行時GPU最大需要的空間 engine = builder.build_engine(network,config)
將鏈接中相應的代碼進行修改或添加,就沒有這個問題了。
到此這篇關于PyTorch模型轉(zhuǎn)TensorRT是怎么實現(xiàn)的?的文章就介紹到這了,更多相關PyTorch模型轉(zhuǎn)TensorRT內(nèi)容請搜索好吧啦網(wǎng)以前的文章或繼續(xù)瀏覽下面的相關文章希望大家以后多多支持好吧啦網(wǎng)!
相關文章:
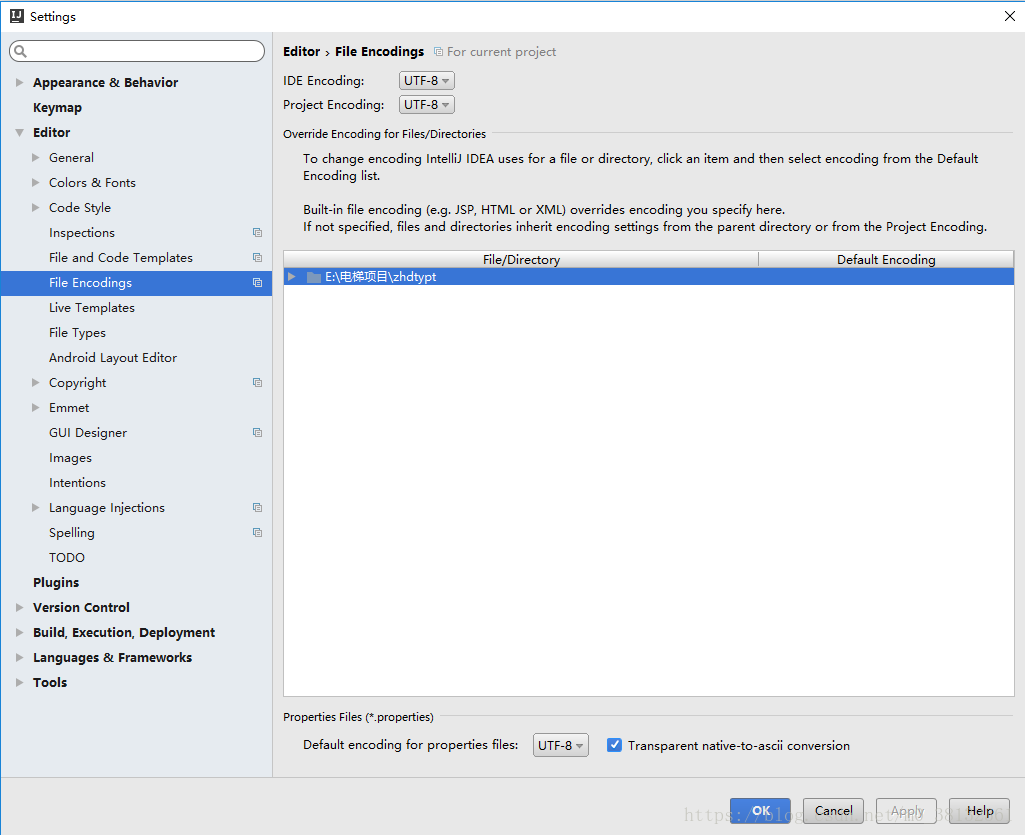