python 基于卡方值分箱算法的實(shí)現(xiàn)示例
原理很簡單,初始分20箱或更多,先確保每箱中都含有0,1標(biāo)簽,對不包含0,1標(biāo)簽的箱向前合并,計(jì)算各箱卡方值,對卡方值最小的箱向后合并,代碼如下
import pandas as pdimport numpy as npimport scipyfrom scipy import statsdef chi_bin(DF,var,target,binnum=5,maxcut=20): ’’’ DF:data var:variable target:target / label binnum: the number of bins output maxcut: initial bins number ’’’ data=DF[[var,target]] #equifrequent cut the var into maxcut bins data['cut'],breaks=pd.qcut(data[var],q=maxcut,duplicates='drop',retbins=True) #count 1,0 in each bin count_1=data.loc[data[target]==1].groupby('cut')[target].count() count_0=data.loc[data[target]==0].groupby('cut')[target].count() #get bins value: min,max,count 0,count 1 bins_value=[*zip(breaks[:maxcut-1],breaks[1:],count_0,count_1)] #define woe def woe_value(bins_value): df_woe=pd.DataFrame(bins_value) df_woe.columns=['min','max','count_0','count_1'] df_woe['total']=df_woe.count_1+df_woe.count_0 df_woe['bad_rate']=df_woe.count_1/df_woe.total df_woe['woe']=np.log((df_woe.count_0/df_woe.count_0.sum())/(df_woe.count_1/df_woe.count_1.sum())) return df_woe #define iv def iv_value(df_woe): rate=(df_woe.count_0/df_woe.count_0.sum())-(df_woe.count_1/df_woe.count_1.sum()) iv=np.sum(rate * df_woe.woe) return iv #make sure every bin contain 1 and 0 ##first bin merge backwards for i in range(len(bins_value)): if 0 in bins_value[0][2:]: bins_value[0:2]=[(bins_value[0][0],bins_value[1][1],bins_value[0][2]+bins_value[1][2],bins_value[0][3]+bins_value[1][3])] continue ##bins merge forwards if 0 in bins_value[i][2:]: bins_value[i-1:i+1]=[(bins_value[i-1][0],bins_value[i][1],bins_value[i-1][2]+bins_value[i][2],bins_value[i-1][3]+bins_value[i][3])] break else: break #calculate chi-square merge the minimum chisquare while len(bins_value)>binnum: chi_squares=[] for i in range(len(bins_value)-1): a=bins_value[i][2:] b=bins_value[i+1][2:] chi_square=scipy.stats.chi2_contingency([a,b])[0] chi_squares.append(chi_square) #merge the minimum chisquare backwards i = chi_squares.index(min(chi_squares)) bins_value[i:i+2]=[( bins_value[i][0], bins_value[i+1][1], bins_value[i][2]+bins_value[i+1][2], bins_value[i][3]+bins_value[i+1][3])]df_woe=woe_value(bins_value) #print bin number and iv print('箱數(shù):{},iv:{:.6f}'.format(len(bins_value),iv_value(df_woe))) #return bins and woe information return woe_value(bins_value)
以下是效果:
初始分成10箱,目標(biāo)為3箱
chi_bin(data,'age','SeriousDlqin2yrs',binnum=3,maxcut=10)
箱數(shù):8,iv:0.184862箱數(shù):7,iv:0.184128箱數(shù):6,iv:0.179518箱數(shù):5,iv:0.176980箱數(shù):4,iv:0.172406箱數(shù):3,iv:0.160015min max count_0 count_1 total bad_rate woe0 0.0 52.0 70293 7077 77370 0.091470 -0.2662331 52.0 61.0 29318 1774 31092 0.057056 0.2429092 61.0 72.0 26332 865 27197 0.031805 0.853755
到此這篇關(guān)于python 基于卡方值分箱算法的實(shí)現(xiàn)示例的文章就介紹到這了,更多相關(guān)python 卡方值分箱算法內(nèi)容請搜索好吧啦網(wǎng)以前的文章或繼續(xù)瀏覽下面的相關(guān)文章希望大家以后多多支持好吧啦網(wǎng)!
相關(guān)文章:
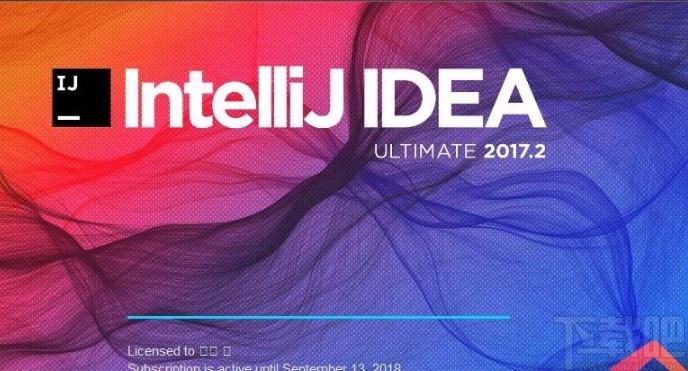