python實現簡單遺傳算法
ObjFunction.py
import mathdef GrieFunc(vardim, x, bound): ''' Griewangk function ''' s1 = 0. s2 = 1. for i in range(1, vardim + 1): s1 = s1 + x[i - 1] ** 2 s2 = s2 * math.cos(x[i - 1] / math.sqrt(i)) y = (1. / 4000.) * s1 - s2 + 1 y = 1. / (1. + y) return ydef RastFunc(vardim, x, bound): ''' Rastrigin function ''' s = 10 * 25 for i in range(1, vardim + 1): s = s + x[i - 1] ** 2 - 10 * math.cos(2 * math.pi * x[i - 1]) return s
GAIndividual.py
import numpy as npimport ObjFunctionclass GAIndividual: ’’’ individual of genetic algorithm ’’’ def __init__(self, vardim, bound): ’’’ vardim: dimension of variables bound: boundaries of variables ’’’ self.vardim = vardim self.bound = bound self.fitness = 0. def generate(self): ’’’ generate a random chromsome for genetic algorithm ’’’ len = self.vardim rnd = np.random.random(size=len) self.chrom = np.zeros(len) for i in xrange(0, len): self.chrom[i] = self.bound[0, i] + (self.bound[1, i] - self.bound[0, i]) * rnd[i] def calculateFitness(self): ’’’ calculate the fitness of the chromsome ’’’ self.fitness = ObjFunction.GrieFunc( self.vardim, self.chrom, self.bound)
GeneticAlgorithm.py
import numpy as npfrom GAIndividual import GAIndividualimport randomimport copyimport matplotlib.pyplot as pltclass GeneticAlgorithm: ’’’ The class for genetic algorithm ’’’ def __init__(self, sizepop, vardim, bound, MAXGEN, params): ’’’ sizepop: population sizepop vardim: dimension of variables bound: boundaries of variables MAXGEN: termination condition param: algorithm required parameters, it is a list which is consisting of crossover rate, mutation rate, alpha ’’’ self.sizepop = sizepop self.MAXGEN = MAXGEN self.vardim = vardim self.bound = bound self.population = [] self.fitness = np.zeros((self.sizepop, 1)) self.trace = np.zeros((self.MAXGEN, 2)) self.params = params def initialize(self): ’’’ initialize the population ’’’ for i in xrange(0, self.sizepop): ind = GAIndividual(self.vardim, self.bound) ind.generate() self.population.append(ind) def evaluate(self): ’’’ evaluation of the population fitnesses ’’’ for i in xrange(0, self.sizepop): self.population[i].calculateFitness() self.fitness[i] = self.population[i].fitness def solve(self): ’’’ evolution process of genetic algorithm ’’’ self.t = 0 self.initialize() self.evaluate() best = np.max(self.fitness) bestIndex = np.argmax(self.fitness) self.best = copy.deepcopy(self.population[bestIndex]) self.avefitness = np.mean(self.fitness) self.trace[self.t, 0] = (1 - self.best.fitness) / self.best.fitness self.trace[self.t, 1] = (1 - self.avefitness) / self.avefitness print('Generation %d: optimal function value is: %f; average function value is %f' % ( self.t, self.trace[self.t, 0], self.trace[self.t, 1])) while (self.t < self.MAXGEN - 1): self.t += 1 self.selectionOperation() self.crossoverOperation() self.mutationOperation() self.evaluate() best = np.max(self.fitness) bestIndex = np.argmax(self.fitness) if best > self.best.fitness: self.best = copy.deepcopy(self.population[bestIndex]) self.avefitness = np.mean(self.fitness) self.trace[self.t, 0] = (1 - self.best.fitness) / self.best.fitness self.trace[self.t, 1] = (1 - self.avefitness) / self.avefitness print('Generation %d: optimal function value is: %f; average function value is %f' % ( self.t, self.trace[self.t, 0], self.trace[self.t, 1])) print('Optimal function value is: %f; ' % self.trace[self.t, 0]) print 'Optimal solution is:' print self.best.chrom self.printResult() def selectionOperation(self): ’’’ selection operation for Genetic Algorithm ’’’ newpop = [] totalFitness = np.sum(self.fitness) accuFitness = np.zeros((self.sizepop, 1)) sum1 = 0. for i in xrange(0, self.sizepop): accuFitness[i] = sum1 + self.fitness[i] / totalFitness sum1 = accuFitness[i] for i in xrange(0, self.sizepop): r = random.random() idx = 0 for j in xrange(0, self.sizepop - 1): if j == 0 and r < accuFitness[j]: idx = 0 break elif r >= accuFitness[j] and r < accuFitness[j + 1]: idx = j + 1 break newpop.append(self.population[idx]) self.population = newpop def crossoverOperation(self): ’’’ crossover operation for genetic algorithm ’’’ newpop = [] for i in xrange(0, self.sizepop, 2): idx1 = random.randint(0, self.sizepop - 1) idx2 = random.randint(0, self.sizepop - 1) while idx2 == idx1: idx2 = random.randint(0, self.sizepop - 1) newpop.append(copy.deepcopy(self.population[idx1])) newpop.append(copy.deepcopy(self.population[idx2])) r = random.random() if r < self.params[0]: crossPos = random.randint(1, self.vardim - 1) for j in xrange(crossPos, self.vardim): newpop[i].chrom[j] = newpop[i].chrom[ j] * self.params[2] + (1 - self.params[2]) * newpop[i + 1].chrom[j] newpop[i + 1].chrom[j] = newpop[i + 1].chrom[j] * self.params[2] + (1 - self.params[2]) * newpop[i].chrom[j] self.population = newpop def mutationOperation(self): ’’’ mutation operation for genetic algorithm ’’’ newpop = [] for i in xrange(0, self.sizepop): newpop.append(copy.deepcopy(self.population[i])) r = random.random() if r < self.params[1]: mutatePos = random.randint(0, self.vardim - 1) theta = random.random() if theta > 0.5: newpop[i].chrom[mutatePos] = newpop[i].chrom[ mutatePos] - (newpop[i].chrom[mutatePos] - self.bound[0, mutatePos]) * (1 - random.random() ** (1 - self.t / self.MAXGEN)) else: newpop[i].chrom[mutatePos] = newpop[i].chrom[ mutatePos] + (self.bound[1, mutatePos] - newpop[i].chrom[mutatePos]) * (1 - random.random() ** (1 - self.t / self.MAXGEN)) self.population = newpop def printResult(self): ’’’ plot the result of the genetic algorithm ’’’ x = np.arange(0, self.MAXGEN) y1 = self.trace[:, 0] y2 = self.trace[:, 1] plt.plot(x, y1, ’r’, label=’optimal value’) plt.plot(x, y2, ’g’, label=’average value’) plt.xlabel('Iteration') plt.ylabel('function value') plt.title('Genetic algorithm for function optimization') plt.legend() plt.show()
運行程序:
if __name__ == '__main__': bound = np.tile([[-600], [600]], 25) ga = GA(60, 25, bound, 1000, [0.9, 0.1, 0.5]) ga.solve()
作者:Alex Yu出處:http://www.cnblogs.com/biaoyu/
以上就是python實現簡單遺傳算法的詳細內容,更多關于python 遺傳算法的資料請關注好吧啦網其它相關文章!
相關文章:
1. Intellij IDEA 2019 最新亂碼問題及解決必殺技(必看篇)2. JS+css3實現幻燈片輪播圖3. java實現圖形化界面計算器4. 《javascript設計模式》學習筆記三:Javascript面向對象程序設計單例模式原理與實現方法分析5. JS繪圖Flot如何實現動態可刷新曲線圖6. IntelliJ IDEA設置條件斷點的方法步驟7. 未來的J2EE主流應用框架:對比Spring和EJB38. ASP.NET MVC獲取多級類別組合下的產品9. 關于HTML5的img標簽10. 原生js XMLhttprequest請求onreadystatechange執行兩次的解決
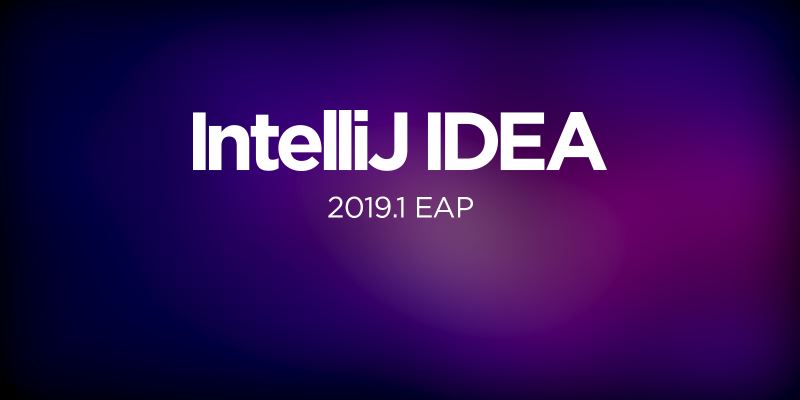