python數(shù)據(jù)分析之用sklearn預(yù)測(cè)糖尿病
本數(shù)據(jù)集內(nèi)含十個(gè)屬性列
Pergnancies: 懷孕次數(shù)
Glucose:血糖濃度
BloodPressure:舒張壓(毫米汞柱)
SkinThickness:肱三頭肌皮膚褶皺厚度(毫米)
Insulin:兩個(gè)小時(shí)血清胰島素(μU/毫升)
BMI:身體質(zhì)量指數(shù),體重除以身高的平方
Diabets Pedigree Function: 疾病血統(tǒng)指數(shù)
是否和遺傳相關(guān),Height:身高(厘米)
Age:年齡
Outcome:0表示不患病,1表示患病。
任務(wù):建立機(jī)器學(xué)習(xí)模型以準(zhǔn)確預(yù)測(cè)數(shù)據(jù)集中的患者是否患有糖尿病
二、準(zhǔn)備工作查閱資料得知各屬性的數(shù)據(jù)值要求,方面后期對(duì)于數(shù)據(jù)的分析與處理過(guò)程。
屬性列名稱 數(shù)據(jù)值要求
Pergnancies(懷孕次數(shù)) 符合常理即可(可為0)
Glucose(血糖濃度) 正常值為:80~120
BloodPressure(舒張壓(毫米汞柱)) 正常值為:60~80
SkinThickness(肱三頭肌皮膚褶皺厚度(毫米)) 不為0
Insulin(兩個(gè)小時(shí)血清胰島素(/毫升)) 正常值為:35~145
BMI(身體質(zhì)量指數(shù):體重除以身高的平方) 正常值為:18.5~24.9
Diabets Pedigree Function:(疾病血統(tǒng)指數(shù):是否和遺傳相關(guān)) 無(wú)特殊值要求
Height(身高(厘米)) 不為0 符合常理即可
Age(年齡) 符合常理即可
Outcome(0表示不患病,1表示患病) 標(biāo)簽值
三、實(shí)驗(yàn)環(huán)境和工具python3.5.6 + jupyter
數(shù)據(jù)處理 pandas、numpy
可視化 matplotlib、seaborn
模型構(gòu)建 sklearn
四、預(yù)測(cè)分析4.1探索性數(shù)據(jù)分析數(shù)據(jù)描述
首先觀察基本的數(shù)據(jù)類型,以及數(shù)據(jù)是否存在缺失情況,簡(jiǎn)要統(tǒng)計(jì)信息
all_data.shapeall_data.info()
<class ’pandas.core.frame.DataFrame’>RangeIndex: 768 entries, 0 to 767Data columns (total 10 columns): # Column Non-Null Count Dtype --- ------ -------------- ----- 0 Pregnancies 768 non-null int64 1 Glucose 768 non-null int64 2 BloodPressure 768 non-null int64 3 SkinThickness 768 non-null int64 4 Insulin 768 non-null int64 5 BMI 768 non-null float64 6 DiabetesPedigreeFunction 768 non-null float64 7 Age 768 non-null int64 8 Height 766 non-null object 9 Outcome 768 non-null int64 dtypes: float64(2), int64(7), object(1)memory usage: 60.1+ KB
數(shù)據(jù)總量時(shí)比較少的只有768個(gè)例子,可以看到除Height外的屬性都為數(shù)值型屬性。在后續(xù)數(shù)據(jù)預(yù)處理過(guò)程需要對(duì)Height屬性進(jìn)行類型轉(zhuǎn)換操作。目前沒(méi)有缺失值的出現(xiàn)。
# height 數(shù)值類型 為object 需要轉(zhuǎn)化為 數(shù)值型all_data = all_data.astype({’Height’:’float64’})
all_data.describe()
發(fā)現(xiàn)兩個(gè)問(wèn)題:
1.缺失值
從其中的min值可以很直觀地觀察到,Glucose, BloodPressure, SkinTinckness, Insulin, BMI等特征存在0值的情況(當(dāng)然Pregnancies根據(jù)常識(shí)判斷是可以為0的)。而根據(jù)常規(guī)范圍明顯可以判定這些0值是不合理的,所以也是一種缺失值缺失值,后續(xù)數(shù)據(jù)預(yù)處理需要對(duì)這些缺失值進(jìn)行填充處理。
2.離群值/異常值
Glucose,BloodPressure,SkinTinckness,Insulin等特征的max值和75%分位點(diǎn)值或者min值和25%分位點(diǎn)值之間的差距比較大,初步判斷可能存在離群值/異常值。尤其是SkinThickness和Insulin特征(具體見(jiàn)圖4紅色框部分),后續(xù)可以通過(guò)可視化進(jìn)一步直觀地觀察判斷。
為了方便后序?qū)θ笔е档慕y(tǒng)一處理,將異常值統(tǒng)一替換為np.nan。
import numpy as np#缺失值替換 經(jīng)分析,除懷孕次數(shù),其他特征的0值表示缺失值 替換為np.nanreplace_list = [’Glucose’, ’BloodPressure’, ’SkinThickness’, ’Insulin’, ’BMI’, ’Height’]all_data.loc[:,replace_list] = all_data.loc[:,replace_list].replace({0:np.nan})
#各特征缺失數(shù)量統(tǒng)計(jì)null_count = all_data.isnull().sum().values# 缺失值情況plt.figure()sns.barplot(x = null_count, y = all_data.columns)for x, y in enumerate(null_count): plt.text(y, x, '%s' %y, horizontalalignment=’center’, verticalalignment=’center’)plt.show()
可以觀察到Glucose,Insulin,SkinThickness,BMI,Height等特征都存在缺失值。并且 Insulin,SkinThickness缺失值比較多,分別占到了48%,30%的比例。所以后期數(shù)據(jù)預(yù)處理也是很關(guān)鍵的。
五、可視化分析接下來(lái)通過(guò)更多針對(duì)性的可視化,來(lái)進(jìn)一步探索特征值的分布以及特征和預(yù)測(cè)變量之間的關(guān)系
# 患病和不患病情況下 箱線圖查看數(shù)據(jù)分散情況for col in all_data.columns: plt.figure(figsize = (10,6)) if all_data[col].unique().shape[0] > 2:sns.boxplot(x='Outcome', y=col, data=all_data.dropna()) else:sns.countplot(col,hue = ’Outcome’,data = all_data.dropna()) plt.title(col) plt.show()
部分輸出:
觀察患病和不患病情況下 各特征值或者人數(shù)分布label接近2:1 存在一定的分布不平衡 像insulin之類的特征離群值是比較多的,由于離群值會(huì)對(duì)模型評(píng)估產(chǎn)生影響,所以后續(xù)可能要做處理,剔除偏離較大的離群值
# 患病和不患病情況下 各特征的分布情況for col in all_data.drop(’Outcome’,1).columns: plt.figure() sns.displot(data = all_data, x = col,hue = ’Outcome’,kind=’kde’) plt.show()
部分輸出:
1.從數(shù)據(jù)樣本本身出發(fā)研究數(shù)據(jù)分布特征,可以發(fā)現(xiàn)在患病和不患病兩種情況下,部分特征的密度分布比較相近,特別是height的分布圖,發(fā)現(xiàn)兩曲線基本相近。感覺(jué)和label之間的相關(guān)性都不是很強(qiáng)。
2.同時(shí),可以發(fā)現(xiàn)部分特征存在右偏的現(xiàn)象(skewness (偏度) 描述數(shù)據(jù)分布形態(tài)的統(tǒng)計(jì)量,其描述的是某總體取值分布的對(duì)稱性),考慮到需要數(shù)據(jù)盡量服從正態(tài)分布,所以后續(xù)數(shù)據(jù)預(yù)處理需要對(duì)存在一定偏度的特征進(jìn)行相關(guān)處理。
# 觀察各特征分布和患病的關(guān)系corr = all_data.corr()plt.figure(figsize = (8,6))sns.heatmap(corr,annot = True,cmap = ’Blues’)plt.show()
heatmap()函數(shù)可以直觀地將數(shù)據(jù)值的大小以定義的顏色深淺表示出來(lái)。
1.可以發(fā)現(xiàn)顏色相對(duì)來(lái)說(shuō)都比較淺,也就是說(shuō)無(wú)論是特征和特征之間還是特征和outcome標(biāo)簽之間的相關(guān)性都沒(méi)有很高。
2.發(fā)現(xiàn)其余各特征變量中與outcome的相關(guān)度中最高的是Glucose 屬性值為0.49,最低的是Height屬性值為0.059。
3.同時(shí)觀察特征與特征之間的關(guān)系,發(fā)現(xiàn)Insulin與Glucose,BMI和SkinThickness之間的相關(guān)度分別為0.58,0.65屬于比較高的相關(guān)性,由于Insulin是一個(gè)確實(shí)比較嚴(yán)重的特征,而相關(guān)性可以是一種協(xié)助填充缺失值的方法。
plt.figure()sns.scatterplot(x = ’Insulin’, y = ’Glucose’, data = all_data)plt.show()sns.scatterplot(x = ’Insulin’, y = ’BMI’, data = all_data)plt.show()sns.scatterplot(x = ’Insulin’, y = ’Age’, data = all_data)plt.show()plt.figure()sns.scatterplot(x = ’SkinThickness’, y = ’BMI’, data = all_data)plt.show()sns.scatterplot(x = ’SkinThickness’, y = ’Glucose’, data = all_data)plt.show()sns.scatterplot(x = ’SkinThickness’, y = ’BloodPressure’, data = all_data)plt.show()
部分輸出:
因?yàn)闆Q策樹(shù)幾乎不需要數(shù)據(jù)預(yù)處理。其他方法經(jīng)常需要數(shù)據(jù)標(biāo)準(zhǔn)化,創(chuàng)建虛擬變量和刪除缺失值。
# 讀取數(shù)據(jù)all_data = pd.read_csv(’data.csv’)# height 數(shù)值類型 為object 需要轉(zhuǎn)化為 數(shù)值型all_data = all_data.astype({’Height’:’float64’})# all_data.dropna(inplace = True)# 特征feature_data = all_data.drop(’Outcome’,1)# 標(biāo)簽label = all_data[’Outcome’]base_model = DecisionTreeClassifier()base_scores = cross_validate(base_model, feature_data, label,cv=5,return_train_score=True)print(base_scores[’test_score’].mean())
0.6954248366013072
七、數(shù)據(jù)預(yù)處理綜合前面分析,先做了以下處理
# 讀取數(shù)據(jù)all_data = pd.read_csv(’data.csv’)# height 數(shù)值類型 為object 需要轉(zhuǎn)化為 數(shù)值型all_data = all_data.astype({’Height’:’float64’})# 理論缺失值0替換為np.nanreplace_list = [’Glucose’, ’BloodPressure’, ’SkinThickness’, ’Insulin’, ’BMI’, ’Height’]all_data.loc[:,replace_list] = all_data.loc[:,replace_list].replace({0:np.nan})# 刪除相關(guān)性低的Heightall_data.drop(’Height’,1,inplace = True)八、離群值處理
1.經(jīng)過(guò)前面的分析發(fā)現(xiàn)數(shù)據(jù)是存在部分離群值的,雖然實(shí)驗(yàn)本身就是關(guān)于疾病預(yù)測(cè),異常值的存在屬于正常現(xiàn)象。但是對(duì)于一些可能超出人體接受范圍的值,衡量對(duì)預(yù)測(cè)的影響之后,由于數(shù)據(jù)量比較小,這里選擇刪除極端異常點(diǎn)。
2.極端異常點(diǎn) :上限的計(jì)算公式為Q3+3(Q3-Q1) 下界的計(jì)算公式為Q1-3(Q3-Q1))。
# remove the outliers# 異常點(diǎn) 上須的計(jì)算公式為Q3+1.5(Q3-Q1);下須的計(jì)算公式為Q1-1.5(Q3-Q1)# 極端異常點(diǎn) :上限的計(jì)算公式為Q3+3(Q3-Q1) 下界的計(jì)算公式為Q1-3(Q3-Q1)# 由于數(shù)據(jù)量比較少 所以選擇刪除極端異常值def remove_outliers(feature,all_data): first_quartile = all_data[feature].describe()[’25%’] third_quartile = all_data[feature].describe()[’75%’] iqr = third_quartile - first_quartile # 異常值下標(biāo) index = all_data[(all_data[feature] < (first_quartile - 3*iqr)) | (all_data[feature] > (first_quartile + 3*iqr))].index all_data = all_data.drop(index) return all_dataoutlier_features = [’Insulin’, ’Glucose’, ’BloodPressure’, ’SkinThickness’, ’BMI’, ’DiabetesPedigreeFunction’]for feat in outlier_features: all_data = remove_outliers(feat,all_data)
處理之后的數(shù)據(jù)基本的統(tǒng)計(jì)信息
1.直接刪除處理
def drop_method(all_data): median_fill = [’Glucose’, ’BloodPressure’,’SkinThickness’, ’BMI’,’Height’] for column in median_fill:median_val = all_data[column].median()all_data[column].fillna(median_val, inplace=True) all_data.dropna(inplace = True) return all_data
2.中值填充
def median_method(): for column in list(all_data.columns[all_data.isnull().sum() > 0]):median = all_data[column].median()all_data[column].fillna(median, inplace=True)
3.KNNImputer填充
def knn_method(): # 先將缺失值比較少的特征用中值填充 values = {’Glucose’: all_data[’Glucose’].median(),’BloodPressure’:all_data[’BloodPressure’].median(),’BMI’:all_data[’BMI’].median()} all_data.fillna(value=values,inplace=True) # 用KNNImputer 填充 Insulin SkinThickness corr_SkinThickness = [’BMI’, ’Glucose’,’BloodPressure’, ’SkinThickness’] # 權(quán)重按距離的倒數(shù)表示。在這種情況下,查詢點(diǎn)的近鄰比遠(yuǎn)處的近鄰具有更大的影響力 SkinThickness_imputer = KNNImputer(weights = ’distance’) all_data[corr_SkinThickness] = SkinThickness_imputer.fit_transform(all_data[corr_SkinThickness]) corr_Insulin = [’Glucose’, ’BMI’,’BloodPressure’, ’Insulin’] Insulin_imputer = KNNImputer(weights = ’distance’) all_data[corr_Insulin] = Insulin_imputer.fit_transform(all_data[corr_Insulin])
4.隨機(jī)森林填充
from sklearn.ensemble import RandomForestRegressorfrom sklearn.impute import SimpleImputer # 用來(lái)填補(bǔ)缺失值def predict_method(feature): # 復(fù)制一份數(shù)據(jù) 避免對(duì)原數(shù)據(jù)做出不必要的修改 copy_data = all_data.copy() # 缺失了的下標(biāo) predict_index = copy_data[copy_data[feature].isnull()].index # 沒(méi)缺失的下標(biāo) train_index = copy_data[feature].dropna().index # 用作預(yù)測(cè) 的訓(xùn)練集標(biāo)簽 train_label = copy_data.loc[train_index,feature] copy_data = copy_data.drop(feature,axis=1) # 對(duì)特征先用中值填充 imp_median = SimpleImputer(strategy=’median’) # 用作預(yù)測(cè)的訓(xùn)練集特征 train_feature = copy_data.loc[train_index] train_feature = imp_median.fit_transform(train_feature) # 需要進(jìn)行預(yù)測(cè)填充處理的缺失值 pre_feature = copy_data.loc[predict_index] pre_feature = imp_median.fit_transform(pre_feature) # 選取隨機(jī)森林模型 fill_model = RandomForestRegressor() fill_model = fill_model.fit(train_feature,train_label) # 預(yù)測(cè) 填充 pre_value = fill_model.predict(pre_feature) all_data.loc[predict_index,feature] = pre_value#用隨機(jī)森林的方法填充缺失值較多的 SkinThickness 和 Insulin 缺失值predict_method('Insulin')predict_method('SkinThickness')# 其余值中值填充for column in list(all_data.columns[all_data.isnull().sum() > 0]): median = all_data[column].median() all_data[column].fillna(median, inplace=True)十、特征工程
# 特征feture_data = all_data.drop(’Outcome’,1)# 標(biāo)簽label = all_data[’Outcome’]
# 利用BMI和身高構(gòu)造weight特征# BMI = weight(kg) / height(m)**2feture_data[’weight’] = (0.01*feture_data[’Height’])**2 * feture_data[’BMI’]十一、數(shù)據(jù)標(biāo)準(zhǔn)化
# 標(biāo)準(zhǔn)化Std = StandardScaler()feture_data = Std.fit_transform(feture_data)十二、模型構(gòu)建與調(diào)參優(yōu)化
用到的模型
from sklearn.svm import SVC,SVRfrom sklearn.tree import DecisionTreeClassifierfrom sklearn.linear_model import LogisticRegressionfrom sklearn.ensemble import RandomForestClassifier,StackingClassifier
調(diào)參方法
from sklearn.model_selection import GridSearchCV
評(píng)估指標(biāo) Accuracy roc_auc
from sklearn.metrics import make_scorer from sklearn.metrics importaccuracy_score from sklearn.metrics import roc_auc_score
def train(model, params): grid_search = GridSearchCV(estimator = model, param_grid = params,scoring=scores,refit=’Accuracy’) grid_search.fit(feture_data,label) print(grid_search.best_estimator_) return grid_searchdef paint(x,y): plt.figure() sns.lineplot(x=x,y=y) plt.show()
SVC
#調(diào)參時(shí)先嘗試一個(gè)大范圍,確定比較小的范圍,然后在小范圍里搜索model = SVC()params = {’C’:np.linspace(0.1, 2, 100)}SVC_grid_search = train(model,params)paint([x for x in range(100)],SVC_grid_search.cv_results_[’mean_test_Accuracy’])paint([x for x in range(100)],SVC_grid_search.cv_results_[’mean_test_AUC’])print('test_Accuracy : {}ntest_AUC : {}'.format(SVC_grid_search.cv_results_[’mean_test_Accuracy’].mean(),SVC_grid_search.cv_results_[’mean_test_AUC’].mean()))
LogisticRegression
model = LogisticRegression()params = {'C':np.linspace(0.1,2,100)}LR_grid_search = train(model,params)paint([x for x in range(100)],LR_grid_search.cv_results_[’mean_test_Accuracy’])paint([x for x in range(100)],LR_grid_search.cv_results_[’mean_test_AUC’])print('test_Accuracy : {}ntest_AUC : {}'.format(LR_grid_search.cv_results_[’mean_test_Accuracy’].mean(),LR_grid_search.cv_results_[’mean_test_AUC’].mean()))
RandomForestClassifier
model = RandomForestClassifier()params = {'n_estimators':[x for x in range(30,50,2)],’min_samples_split’:[x for x in range(4,10)]}RFC_grid_search = train(model,params)print('test_Accuracy : {}ntest_AUC : {}'.format(RFC_grid_search.cv_results_[’mean_test_Accuracy’].mean(),RFC_grid_search.cv_results_[’mean_test_AUC’].mean()))
StackingClassifier
estimators = [ (’SVC’,SVC_grid_search.best_estimator_), (’NB’, LR_grid_search.best_estimator_), (’RFC’, RFC_grid_search.best_estimator_)]model = StackingClassifier(estimators=estimators, final_estimator=SVC())model_score = cross_validate(model,feture_data, label,scoring=scores)print('test_Accuracy : {}ntest_AUC : {}'.format(model_score[’test_Accuracy’].mean(),model_score[’test_AUC’].mean()))
SVC預(yù)測(cè)結(jié)果:
1.直接刪除缺失值以及異常值刪除公式上限Q3+1.5(Q3-Q1);下限計(jì)算公式為Q1-1.5(Q3-Q1)
SVC(C=1.097979797979798)test_Accuracy : 0.8549075391180654test_AUC : 0.511601411290322
2.直接刪除缺失值以及異常值刪除公式上限Q3+3(Q3-Q1);下限計(jì)算公式為Q1-3(Q3-Q1)
SVC(C=1.405050505050505)test_Accuracy : 0.7953321596244133test_AUC : 0.7133755225726653
3.中值填充以及異常值刪除公式上限Q3+3(Q3-Q1);下限計(jì)算公式為Q1-3(Q3-Q1)
SVC(C=1.7888888888888888)test_Accuracy : 0.7814101086443079test_AUC : 0.7248522348166069
1.一些刪除數(shù)據(jù)值的處理方法導(dǎo)致樣本標(biāo)簽的不均衡會(huì)導(dǎo)致對(duì)比例大的樣本造成過(guò)擬合,也就是說(shuō)預(yù)測(cè)偏向樣本數(shù)較多的分類。這樣就會(huì)大大降低模型的泛化能力。表現(xiàn)在準(zhǔn)確率很高,但roc_auc_score很低。上面SVC的預(yù)測(cè)結(jié)果就很好的說(shuō)明了。
2.可以看出由于缺失值比較多,所以反而各種填充方法的效果比直接刪除的效果是要更差的(也有可能我沒(méi)找到合適的填充方法)
3.關(guān)于離群值的處理,主要方法有直接刪除法,替換為缺失值處理,以及中值填充法等。由于缺失值處理那里的效果不是很理想,所以就選擇了直接刪除,并且在平衡了roc_auc_score和accuracy兩個(gè)指標(biāo)后,選擇只刪除極端異常點(diǎn)。
4.關(guān)于樣本0/1比例的問(wèn)題,可以考慮上采樣或者下采樣的方法平衡樣本。本文不涉及。
到此這篇關(guān)于python數(shù)據(jù)分析之用sklearn預(yù)測(cè)糖尿病的文章就介紹到這了,更多相關(guān)用sklearn預(yù)測(cè)糖尿病內(nèi)容請(qǐng)搜索好吧啦網(wǎng)以前的文章或繼續(xù)瀏覽下面的相關(guān)文章希望大家以后多多支持好吧啦網(wǎng)!
相關(guān)文章:
1. ASP動(dòng)態(tài)網(wǎng)頁(yè)制作技術(shù)經(jīng)驗(yàn)分享2. jsp文件下載功能實(shí)現(xiàn)代碼3. asp.net core項(xiàng)目授權(quán)流程詳解4. 在JSP中使用formatNumber控制要顯示的小數(shù)位數(shù)方法5. CSS3實(shí)現(xiàn)動(dòng)態(tài)翻牌效果 仿百度貼吧3D翻牌一次動(dòng)畫(huà)特效6. XMLHTTP資料7. ASP常用日期格式化函數(shù) FormatDate()8. html中的form不提交(排除)某些input 原創(chuàng)9. CSS3中Transition屬性詳解以及示例分享10. ASP基礎(chǔ)入門(mén)第八篇(ASP內(nèi)建對(duì)象Application和Session)
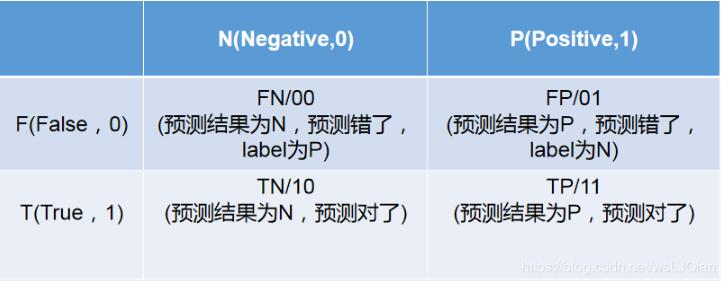